Durable and reliable machines thanks to collaborative artificial intelligence
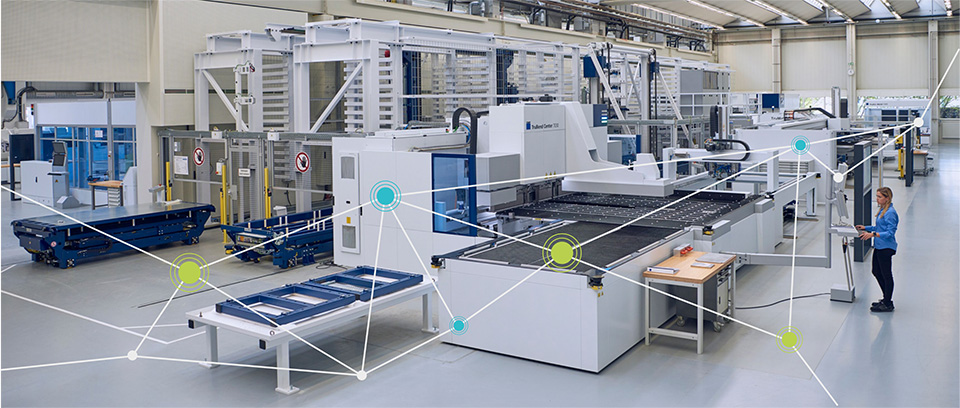
ProKInect
When it comes to improving automated condition monitoring and, in turn, boosting reliability and machine availability, the challenge lies in increasing cooperation between component and machine manufacturers. The data generated during operation is often only available to one of the parties for the purpose of analyzing the machine and component behavior. Furthermore, when AI-based condition monitoring methods are used, the systems sometimes have to be trained on huge volumes of data. This involves creating the necessary technical basis for AI training, data exchange and the aggregation of data across the various hierarchical levels and different production machinery communication protocols. At the same time, secure data storage, shared data spaces and access authorizations are required to ensure the protection and appropriate use of sensitive data. Without compromising their data sovereignty, component and machine manufacturers must be given the ability to decide for themselves in what way their proprietary data can be used by other stakeholders and how data may be used in general.
“ProKInect” is a research project of the German Federal Ministry of Education and Research (BMBF) that aims to increase the availability and reliability of production machinery by implementing a form of automated condition monitoring that relies on distributed, cross-company AI agents. An immediate increase in machine availability can be achieved by preventing the kind of unscheduled downtime that stems from the historic lack of automated methods for detecting machine or component faults. Using the example of a track-guided laser cutting system, a cross-manufacturer form of condition detection is to be implemented as part of the project, along with automated condition monitoring and a predictive maintenance concept. The aim here is to perform condition diagnostics and predict probabilities of failure within the complete system by cooperating in the evaluation of proprietary operating data that starts off fragmented. The successful completion of “ProKInect” will create the necessary technical basis so that data and information can be exchanged on a cross-manufacturer basis between distributed AI agents at the sensor, component, machine and server levels. Within this context, the individual AI agents will be responsible for specific condition monitoring tasks and will implement a hybrid AI approach that uses both knowledge-based and data-based AI models for condition detection and analysis. The AI agents are being trained on a machine-level server with shared, protected data spaces.
Through its ProKInect research work, the Fraunhofer Institute for Structural Durability and System Reliability LBF is improving the clarity and comprehensibility of AI systems in the area of condition monitoring and predictive maintenance. The primary aim is to take expert knowledge concerning failure-induced symptoms, causal relationships and ongoing maintenance and to systematically convert this into transparent AI models in a seamless and automated manner. The expert knowledge of relevance to condition monitoring is being converted into knowledge-based probabilistic causal models that allow the differential diagnosis of various fault causes on the basis of the symptoms and additional information recorded. To facilitate ongoing condition diagnostics, Fraunhofer LBF is developing hybrid AI models that are based on the network structures obtained from the probabilistic causal models and feature the same levels of trainability and performance as artificial neural networks.
With its cross-manufacturer and collaborative approach to condition monitoring, ProKInect is making it possible to visualize the complex causal relationships and fault mechanisms encountered in machine tools in a user-friendly way. In turn, this will be of equal benefit to component and machine manufacturers, as well as the companies operating the systems and the people who use them, by allowing them to detect incipient wear, abnormal behavior or critical machine conditions at an early stage and take proactive steps to prevent machine failures. Within this context, service engineers will likewise be given the opportunity to enter annotated data and information from maintenance situations directly.
»The process of creating a future open platform for condition monitoring in the context of ProKInect is based on multilateral cross-manufacturer cooperation. One of the central elements is an open approach to data exchange that goes beyond the confines of conventional bilateral partnerships.«
Dr.-Ing. Alexander Keck, Maschinendynamik, TRUMPF Werkzeugmaschinen GmbH + Co. KG
A BMBF research project under the “Learning Production Technology — Use of Artificial Intelligence (AI) in Production” (ProLern) initiative undertaken as part of the research program titled “Innovationen für die Produktion, Dienstleistung und Arbeit von morgen” (“Innovation for tomorrow’s production, services and jobs)”.
Research project: Herstellerübergreifende und verteilte KI-Agenten zur Zustandsüberwachung in Werkzeugmaschinen (Cross-manufacturer and distributed AI agents for condition monitoring in machine tools) (ProKInect)
Grant number: 02P20A091
Duration: August 1, 2021 to July 31, 2024
Project partners: TRUMPF Werkzeugmaschinen GmbH + Co. KG, WITTENSTEIN, Tvarit GmbH